Research
1) A computational account of topography in the occipitotemporal cortex via domain-general pressures:
Collaborators: Talia Konkle
Doshi, F. R., & Konkle, T. (2023). Cortical topographic motifs emerge in a self-organized map of object space. Science Advances, 9(25), eade8187.
Doshi, F. R., & Konkle, T. (2023). Face-deprived networks show distributed but not clustered face-selective maps. Journal of Vision, 23(9), 5435-5435.
Doshi, F., & Konkle, T. (2021). Organizational motifs of cortical responses to objects emerge in topographic projections of deep neural networks. Journal of Vision, 21(9), 2226-2226.
Link to talk presented at the Vision Sciences Society 2021 Conference
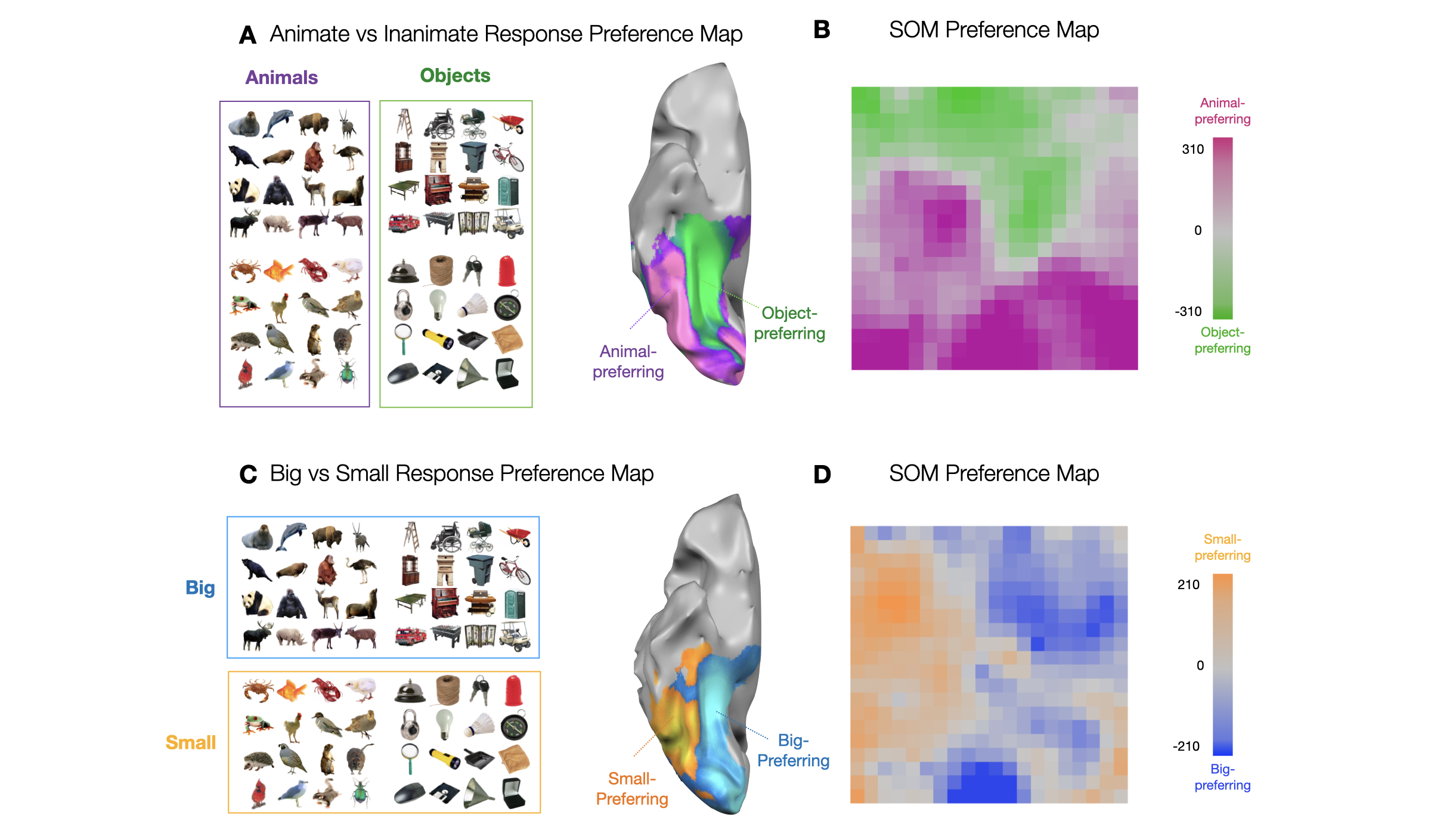
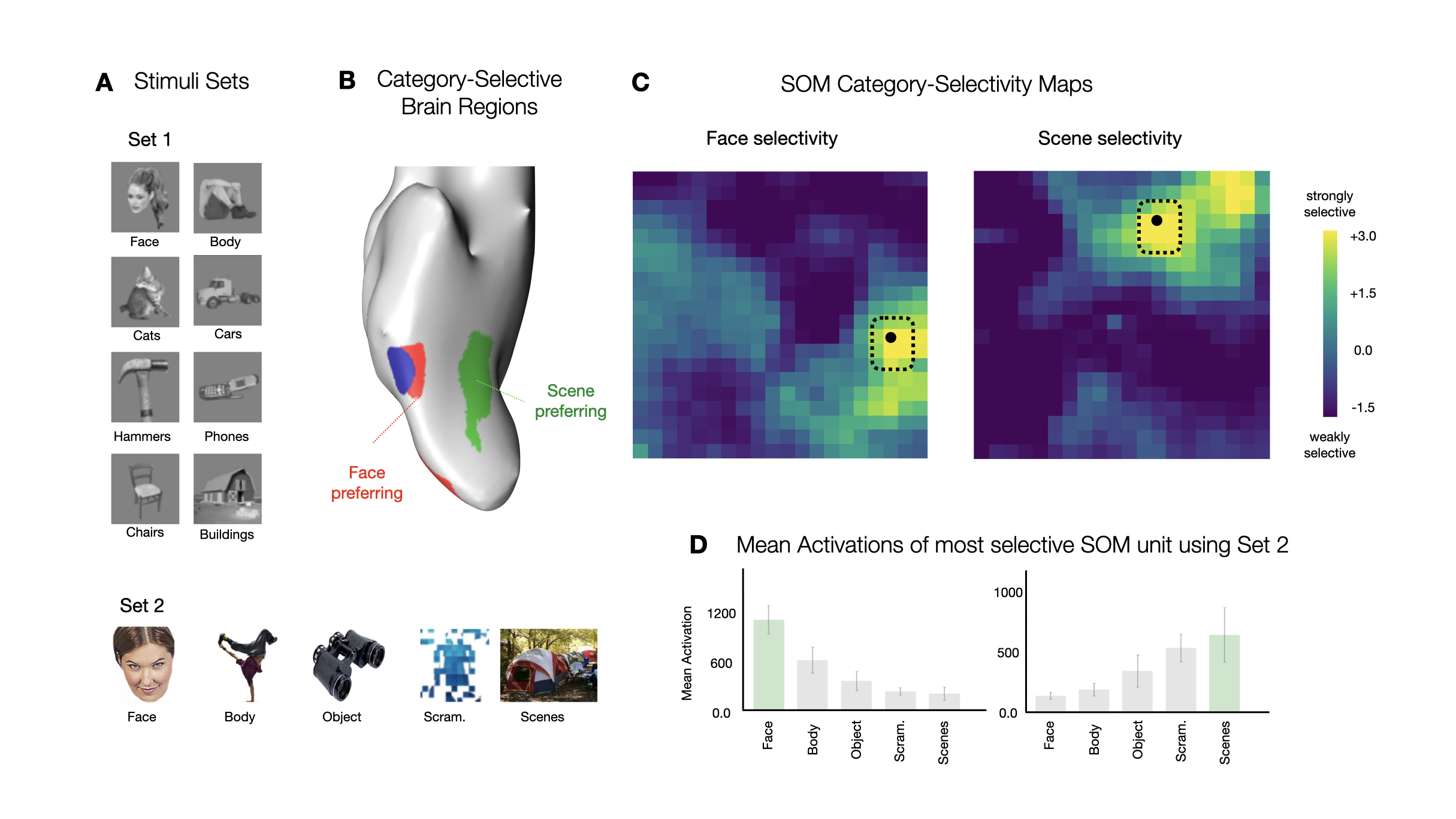
2) Mechanisms of Contour Integration in Humans and Machines:
Collaborators: Talia Konkle and George Alvarez
Doshi, F. R., Konkle, T., & Alvarez, G.A. (2024). A feedforward mechanism for human-like contour integration. Biorxiv, 2024.
Doshi, F. R., Konkle, T., & Alvarez, G.A. (2023). Feedforward Neural Networks can capture Human-like Perceptual and Behavioral Signatures of Contour Integration. In Cognitive Computational Neuroscience (CCN), 2023.
Doshi, F., Konkle, T., & Alvarez, G.A. (2022). Human-like signatures of contour integration in deep neural networks.
Link to talk presented at the Vision Sciences Society 2022 Conference
3) Configural Shape Perception in Deep Neural Networks
Collaborators: Talia Konkle and George Alvarez
Doshi, F. R., Konkle, T., & Alvarez, G.A. (2024). Configural-Shape Representation in Deep Neural Networks. Paper In Cognitive Computational Neuroscience (CCN), 2024.
Doshi, F. R., Konkle, T., & Alvarez, G.A. (2024). Quantifying the Quality of Shape and Texture in Deep Neural Network Models. In Vision Science Society, 2024
4) Perceptual features as optimal proxies for intuitive physical reasoning:
Collaborators: Colin Conwell and George Alvarez
Conwell, C., Doshi, F., Alvarez, G.A.(2019). Shared Representations of Stability in Humans, Supervised, & Unsupervised Neural Networks. In Shared Visual Representations in Human and Machine Intelligence. SVRHM workshop at NeurIPS 2019.
Conwell, C., Doshi, F., Alvarez, G.A.(2019). Human-Like Judgments of Stability Emerge from Purely Perceptual Features: Evidence from Supervised and Unsupervised Deep Neural Networks. In Proceedings of the 3rd Conference on Cognitive Computational Neuroscience (CCN), 2019.
5) Capacity limits in visual working memory:
Collaborators: Hrag Pailian and George Alvarez
- Doshi, F., Pailian, H., & Alvarez, G. A. (2020). Using Deep Convolutional Neural Networks to Examine the Role of Representational Similarity in Visual Working Memory. Journal of Vision, 20(11), 149-149.
Link to poster walkthrough presented at Vision Sciences Society 2020 Conference
Also check out Hrag’s talk here!
6) Previous work:
Liu, Yc., Wu, H., Mayeshiba, T. et al. (2022). Machine learning predictions of irradiation embrittlement in reactor pressure vessel steels. In NPJ Computational Materials, 2022.
Kanakasabapathy, M., Thirumalaraju, P., Kandula, H., Doshi, F., Sivakumar, A., Kartik, D., Gupta, R., Pooniwala, R., Branda, J., Tsibris, A., Kuritzkes, D., Petrozza, J., Bormann, C., Shafiee H. (2021). Adaptive adversarial neural networks for the analysis of lossy and domainshifted datasets of medical images. In Nature Biomedical Engineering, 2021.
Thirumalaraju, P., Bormann, CL., Kanakasabapathy, M., Doshi, F., Souter, I., Dimitriadis, I., Shafiee, H.(2018). Automated sperm morpshology testing using artificial intelligence. In Fertility and sterility, 2018